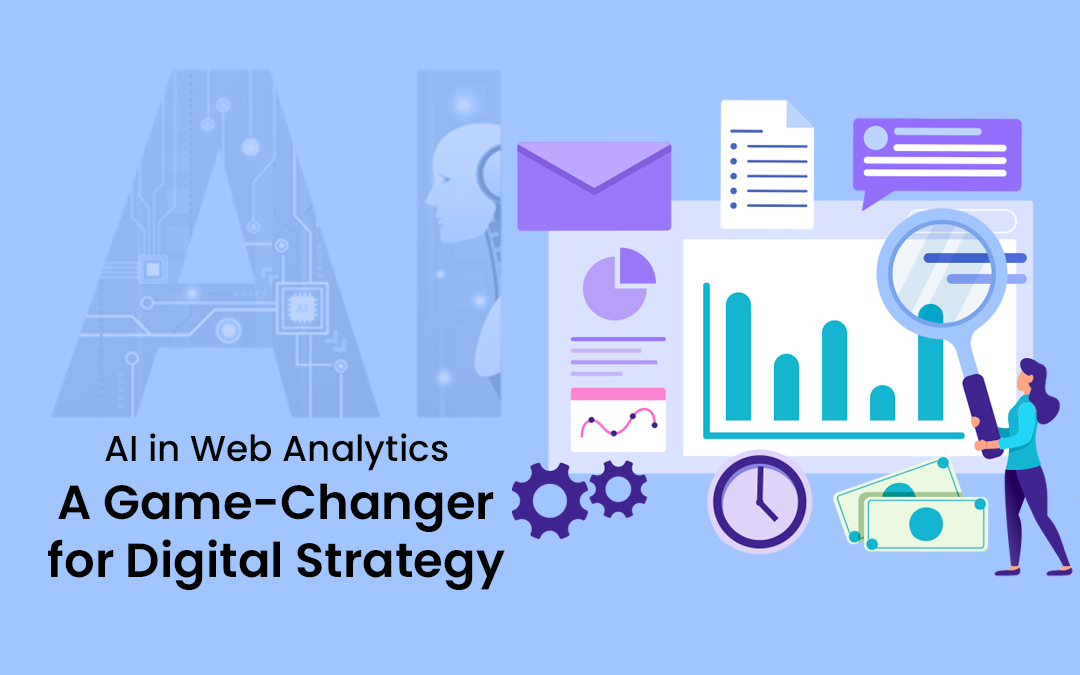
Web analytics has always formed the backbone of monitoring and improving website performance. Conventional analytics has, until recently, focused on the tracking of active users, trends in traffic, and page load times, hence being a cornerstone for any kind of digital strategy. However, with the inclusions of artificial intelligence in this area, web analytics is considering significant evolution and changing the way businesses can use data as a source of competitive advantage.
The Perks of AI-Powered Web Analytics
The introduction of AI into web analytics brings a significant change in how data is processed. AI algorithms can manage large amounts of data quickly, allowing for real-time analysis and instant insights. Businesses can now swiftly adapt to shifts in user behavior and market conditions, enhancing agility and responsiveness.
Predictive analytics: looking ahead
Machine learning models lie at the heart of AI's power, studying past data to predict how users will act and react in the future, along with market trends. Their predictive powers let businesses anticipate customer needs, target marketing campaigns with greater precision, and sharpen retention strategies-all constituents of an asset called data-driven foresight.
Personalization and engagement
AI works quite well in designing personalization of experiences for users based on individual behaviors and preferences for their targeted content and product suggestions. This level of personalization will drive user engagement, customer satisfaction, and ultimately, loyalty.
Automation of insights generation
Automating insights with AI-driven tools is a quantum leap. AI software systems autonomously identifies patterns and anomalies, and key performance indicators, in a way that such analyses are much more efficient and unburden the human analyst to think at higher strategic levels.
Natural language processing put to work
The other area in which AI continues to make notable inroads is natural language processing: NLP helps businesses dissect qualitative data from sources like consumer reviews and social media, giving them a clearer view of user sentiment and brand perception. By doing so, NLP beefs up the ability to better gauge market reactions and surfacing unmet needs.
Advanced segmentation and anomaly detection
AI is advanced segmentation and, further, with high precision, it can go ahead and delineate the groups of users. In this way, there is more appropriate matching with marketing strategies for further resource allocation efficiently. Atypical patterns in web traffic and user behavior are detected in order to identify alerted cases or new opportunities through anomaly detection.
Making ad spend efficient
AI also brings about a much-needed level of advertising budget optimization through the continuous performance data analysis and real-time adjustment of bids to ensure that allocated budget is properly spent to achieve a maximum return on investment.
Visual analytics: intuitive data presentation
Lastly, AI enriches visualization by creating more perceptive and interactive dashboards. Visualization is carried out to assist nontechnical stakeholders and to help them make decisions based on generated knowledge.
Traditional Web Analytics Faces Growing Pains
Understandably, the traditional web analytics has been regarded as the foundation of understanding the performance and user behavior of a website. Its capacity to produce any meaningful interpretations is, though put under question more and more, with a couple of weaknesses being built into its foundation:
- Data overload: Traditional analytics cannot but encounter the overwhelming data generated on multidimensional resources. A sudden outburst of information is likely to hinder its functionality, for this gives rise to information overload almost at every turn.
- Real-time processing: A constraint of traditional tools, which creates lags in understanding user behaviors and market shifts. In such instances, lags cause missed opportunities and sluggish decision-making. Tools in use themselves are a misfit to gain dynamic insights, which otherwise keep changing with evolving user behaviors, since heavy reliance is on static reports and dashboards that rapidly become stale.
- Manual interpretation of data: Traditional analytics require a significant amount of manual labor in interpreting data, the discovery of any patterns, and insight drawing. The process is highly laborious and tending to be really error-prone where humans are involved, depending upon the accuracy and efficiency of the analytics being presented.
- Poor personalization: The personalization in traditional analytics is base-specific and subject-based as it does not implement advanced algorithms. This is a huge limitation that really hampers the effectiveness of personalization.
- Limitations of predictive analysis: Since traditional analytics focus on providing descriptive and diagnostic insights, it falls short in predictive capabilities. In many cases, such tools are not able to predict future concerns and user behaviors.
- Siloed data sources: A large part of traditional analytics operates with siloed data. While fragmented views prevent getting a comprehensive understanding of user interactions, the problem lies in integrating information coming from various channels like websites, social media, mobile apps, etc.
- Dealing with unstructured data: Systems such as traditional ones will not be efficient with unstructured data, such as social media text or user reviews. Besides, the scope of research would be quite narrow.
- Weak anomaly detection: The manual search for anomalies in sizeable data is inconvenient and inefficient. Traditional methods for web analytics may not catch subtle changes that can be a sign of issues or opportunities in the making.
- Scalability: In case business growth is associated with growing information, web analytics instruments usually do not scale decently and, therefore, often work with performance backlogs.
That is why businesses are turning to advanced AI-driven analytic solutions, which have promised to deliver deeper, more actionable insight, to name only a few.
Redefining Web Analytics With AI and Machine Learning
AI is the exhibited intelligence by artificial systems in simulation to human intelligence processes, such as learning, reasoning, and self-correction. An AI system's fundamental objective is to perform various actions that mimic human intelligence, such as visual perception, speech recognition, decision-making, and language translation.
ML is another subset of artificial intelligence, and it is the training of algorithms to learn from data features and make predictions on new ones. A system in ML, with this aspect, does not follow explicit programming but rather learns from examples and makes decisions with minimal human intervention.
AI’s Impact on Web Analytics
AI empowers web analytics through the automation and improvement of data analysis routines, including:
Data processing
- Automation in data collection. Autonomous gathering of data from multiple sources with the use of AI tools leads to more complete and timely datasets.
- Cleaning up data. AI enhances accuracy by identifying and correcting all data errors, such as duplication and wrong entries.
Pattern recognition
- Behavioral analysis. Analysing user behaviour to come up with patterns, such as common navigation paths and most viewed content, to add in user experience.
- Anomaly detection. AI-driven systems detect abnormal traffic patterns and highlight flaws-ill-designed websites or campaigns that are too effective.
Predictive analytics
- Prediction of trends: ML algorithms use historical data to forecast future trends in web traffic and thereby proactively effect changes in strategy.
- Prediction of churn: AI predicts the probability of churn, studying user behavior to run proactive campaigns of user re-engagement.
- Personalized recommendations: AI personalizes recommendations of content based on users' preference, improving customer engagement.
- Dynamic pricing: automatic price optimization with fluctuations in real time, based on user behavior, demand, and market competition to optimize revenue.
Conversion optimization
- A/B testing: the fastest identification of the best-performing designs and strategies for better conversion rates, thanks to the use of AI in automated A/B testing.
- Customer journey mapping: AI maps out a customer journey to identify the point of impact and thus optimize the conversion path.
AI actually turns web analytics from being reactive-descriptive to proactive predictive, driving better decisions for superior performance.
AI-Enhanced Web Analytics Tools
AI powers advanced analytics and predictive capabilities, enabling businesses to make data-driven decisions. A view into some of the best AI-powered web analytics innovators:
Google Analytics 4 (GA4)
Using AI teamed with machine learning, this is the latest in Google analytics, known as GA4, which powers next-generation insights and predictive analytics. Key capabilities: event-based tracking, AI-powered insights, predictive metrics, user-centric data measurement, cross-device tracking, and strong privacy controls.
Adobe Analytics
Adobe Analytics provides an enterprise-class suite to analyze multi-channel customer behavior powered by AI. Key capabilities include AI-powered anomaly detection, predictive analytics, advanced segmentation, cross-channel attribution, real-time data processing, and seamless integrations with other Adobe Experience Cloud products.
Kissmetrics
Kissmetrics focuses on detailed user behavior tracking to measure user engagement, now enhanced with AI capabilities. Core capabilities: Cohort analysis, customer journey mapping, behavioral-based segmentation, funnel analysis, A/B testing insights, and customer retention analytics.
Mixpanel
Mixpanel is a sophisticated tool that works with AI to help enhance user interaction analysis and engagement strategies both for web and mobile applications. Key features: AI-powered predictive analytics, advanced segmentation of users, real-time data visualization, event-based tracking, user retention analysis, and A/B testing capabilities.
Hotjar
Hotjar brings behavior analytics together with AI in assessing user interactions through heatmaps, session recordings, and surveys. Key features: AI-driven heatmaps, session replay analysis, conversion funnels, form analysis, feedback polls, and survey insights.
Decoding User Behavior: AI Innovations
Understanding user behavior is key to digital business. The following analytics tools will light up how users use websites, apps, and other digital platforms in streams of clicks, pages viewed, time spent, and non-content interaction.
Benefits of user behavior insights
- Improved user experience: It can enable the smoothness and enjoyment of the interactions by letting companies detect navigation patterns.
- Driving personalization: With rich insight into users' behaviors, it becomes possible to create tailored content, offers, and recommendations that actually influence engagement and conversion rates.
- Optimizing marketing strategies: This level of detailed analysis in user behavior refines marketing strategies, enhances the targeting of audiences, and ensures resources are optimally allocated for maximum impact.
- Informed product development: Insights into the active actions of users drive product enhancements and prioritization of features, keeping offerings aligned with user needs and preferences.
Real-world examples of AI in web analytics
Netflix
Recommendation by Netflix using AI algorithms helped in suggesting only those content to the user, which matched the interest of an individual viewer. It brought a model that enhanced customer satisfaction and loyalty, and today, more than 80% customers viewing content based on these personalized recommendations.
Spotify
Spotify's 'Discover Weekly' feature uses artificial intelligence to understand users' listening behavior and tastes then creates a playlist for them each week. As a result, it comes out with increased engagement and loyalty from users in the form of this personalized method of discovering music.
Airbnb
Machine learning algorithms at Airbnb analyze patterns in bookings and market trends to recommend listings for optimal pricing. The positive impact on occupancy rates and revenue for hosts is evident, with price competitiveness for guests assured.
Sephora
Sephora has moved toward the use of AI and augmented reality to communicate personalized makeup recommendations and virtual try-ons related to individual consumer preferences. As a result, customer engagement and satisfaction have gone up, while driving in-store and online conversion rates higher.
These examples, in fact, bring out the difficulties of AI in imposing refinement in user behavior insights for a superior user experience, accurate marketing, and improvement in business performance.
Custom vs. Readymade Web Analytics Tools with AI
Criteria | Readymade Web Analytics AI Tools | Custom Web Analytics AI Tools |
---|---|---|
Ease of use | Quick setup and immediate use. Intuitive dashboards for non-technical users. | Tailored to specific business needs, requiring more time to develop. Customizable features but requires development. |
Cost-effectiveness | Generally lower upfront costs. Monthly or annual subscriptions. | Significant investment required for development and deployment. Requires a dedicated team, increasing overall costs. |
Regular updates and support | Frequent updates and feature improvements. Access to support teams and community forums. | Ongoing updates and bug fixes are resource-intensive. Potential for accumulation of technical debt over time. |
Proven reliability | Widely adopted with proven stability. Significant investment in data protection. | Can be optimized for specific use cases but requires continuous monitoring. Customizable security measures and compliance protocols. |
Customization | May not fit specific needs perfectly. Limited to the tool’s predefined capabilities. | Highly relevant insights and functionalities. Developers can modify or add features as needed. |
Scalability | Caps on data volume, user seats, or features. May face issues with high data loads or complex queries. | Designed to handle growing data and user demands. Can be fine-tuned to avoid performance degradation. |
Integration | May have challenges with seamless integration. Dependent on vendor policies and outages. | Can integrate with existing systems and data sources. Achieves a holistic view of data through integration. |
Control and ownership | Dependency on specific vendor's ecosystem. Potential impact from vendor-related issues. | Complete authority over development, deployment, and maintenance. Ability to implement personalized security measures. |
Wrapping Up
Whether AI-driven custom or readymade web analytics tools should be used depends upon the singular needs of your business, resource availability, and strategic objectives. Off-the-shelf tools have speed, cost-efficiency, standard features, and standard support, fitting perfectly for businesses focused on efficiency. On the other hand, custom analytics tools suit organizations with particular needs, deep integrations, and the capacity to invest in tailored solutions that offer greater flexibility and control.
The whole concept of AI and its role in transforming the web analytics space basically voices the need for businesses to stay tuned with technological developments to get the maximum out of innovations. The future of web analytics is now, and it's AI-powered.
Share this post
Leave a comment
All comments are moderated. Spammy and bot submitted comments are deleted. Please submit the comments that are helpful to others, and we'll approve your comments. A comment that includes outbound link will only be approved if the content is relevant to the topic, and has some value to our readers.
Comments (0)
No comment