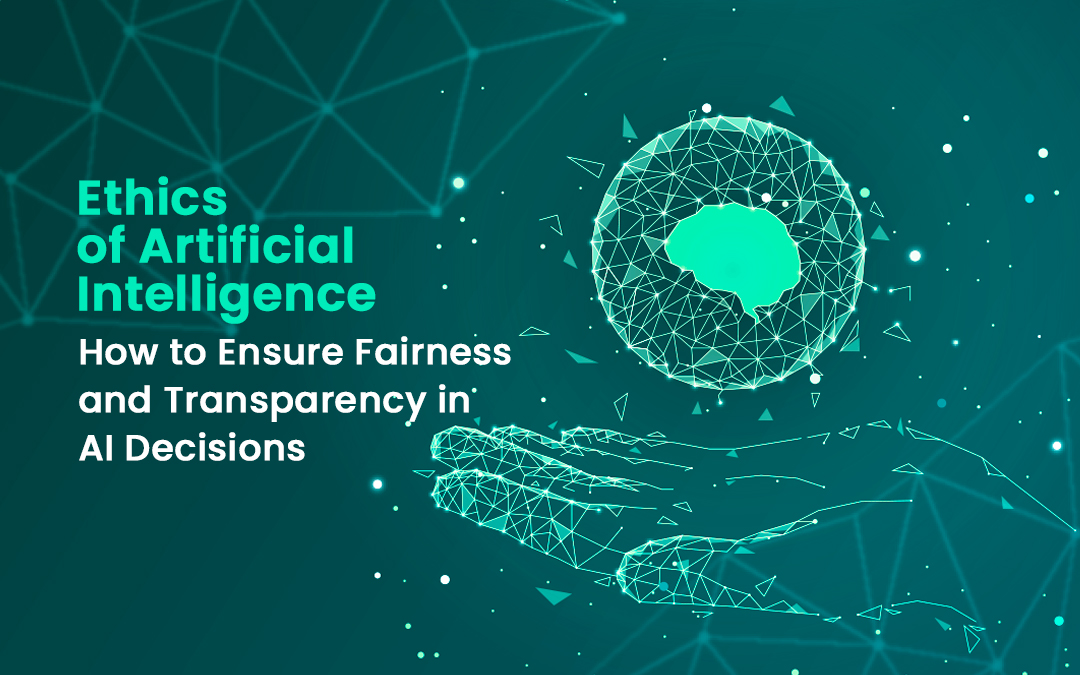
Rapidly changing sectors and redefining our way of life and employment is artificial intelligence (AI). From finance and healthcare to education and entertainment, AI-powered solutions are boosting invention and efficiency in many different fields. Personalized suggestions, autonomous systems, or predictive analytics—all of which reflect AI's impact is growing at hitherto unheard-of rates.
But along with this fast expansion comes the ethical issues raised by artificial intelligence's capacity for decision-making. The possible for biassed or opaque choices poses major ethical concerns as these systems are deployed in increasingly important spheres including law enforcement, hiring procedures, and medical diagnosis. While openness is crucial to inspire confidence in AI-driven systems, ensuring justice in AI choices is crucial to prevent strengthening of society inequities.
Examining the difficulties of bias, responsibility, and the requirement of ethical rules to control AI development and use, this paper will investigate ways to guarantee fairness and transparency in AI decision-making procedures. Solving these problems will help us to create a future in which artificial intelligence systems function fairly and responsibly.
The Task of Preference in Artificial Intelligence
Artificial intelligence is only as good as the data it is fed upon. Built on biassed data, artificial intelligence systems can reinforce and even magnify those prejudices, therefore producing unfair and discriminating results. Recent years have seen a lot of attention paid to this problem since artificial intelligence's influence in hiring, law enforcement, and healthcare has become increasingly important.
Main Causes of AI Bias
AI bias originates mostly from the data used to teach machine learning algorithms. Should historical data show current prejudices—of either institutional, cultural, or social nature—those prejudices are ingrained into the artificial intelligence system. Furthermore, depending on how the algorithms analyze and interpret data, they can induce bias. Biassed results can result from poorly selected features, unbalanced datasets, and lack of varied viewpoints in development teams among other things.
Resources like MIT's analysis of algorithmic bias offer a great summary of how these systems operate and where prejudices could develop, thereby helping one to have a better awareness of the reasons of bias in artificial intelligence.
Biassed AI Decisions: Illustrations from Practical Applications
Already showing negative effects in many real-world applications are biassed artificial intelligence systems. For those with darker skin tones, for example, facial recognition technology have been proven to function poorly, increasing misidentification rates. Most face recognition systems, according to a National Institute of Standards and Technology (NIST) study, have greater error rates for women and people of color, which could result in biassed policing and erroneous arrests (NIST Study on face Recognition).
Another well-known instance is artificial intelligence applied in hiring procedures. While some businesses have used AI technologies to assess job applications, these systems have been shown to favor male applicants for technical roles since they were taught on resumes mostly from men. This kind of prejudice not only prolongs inequality but also keeps qualified applicants off based on pointless criteria. Through Harvard Business Review's analysis of the problem, you can investigate further about artificial intelligence in recruiting prejudices.
Bias's Effects on Marginalized Groups
AI bias disproportionately impacts underprivileged populations, therefore supporting systematic inequality. For instance, biassed algorithms have been shown in healthcare to offer Black patients less quality care suggestions than their White counterparts. Because the algorithms in AI tools meant to prioritize patients for healthcare interventions relied on historical healthcare cost data, which did not account for unequal access to healthcare, a study published in Science revealed that Black patients systematically under-identified as needing more care (Science Study on Bias in Healthcare AI).
Biassed AI tools in law enforcement can lead to overpolicing of some areas. For example, predictive policing systems sometimes depend on past crime data that disproportionately targets low-income neighborhoods, therefore aggravating cycles of inequality. See The Guardian's reporting on predictive policing and artificial intelligence bias for more ideas.
Dealing with artificial intelligence bias is essential to make sure these systems advance justice and equal treatment for all rather than reinforce current inequality.
AI Decision Accountability
The topic of responsibility gets more difficult as artificial intelligence systems participate in high-stakes judgments. Who should answer when an artificial intelligence makes a mistake—such as a misdiagnosis in healthcare or a mistaken legal advice? Assigning responsibility in artificial intelligence systems is challenging since several groups are usually involved, from the companies using the system to the developers designing the algorithm.
In several fields, including finance, law enforcement, and healthcare, artificial intelligence mistakes can have dire results. In the healthcare industry, for instance, AI algorithms used to suggest treatment plans may unintentionally prioritize patients wrongly depending on biassed data, therefore resulting in damage. Predictive policing methods in law enforcement can unfairly target some populations, therefore aggravating systematic inequality. AI-driven loan approval systems in the financial industry can arbitrarily reject applications depending on faulty models or antiquated data.
Explicit lines of responsibility
Clearly defined boundaries of authority help to reduce the possibility of irresponsible AI judgments. Companies implementing artificial intelligence must make sure that there are established responsibility systems that precisely point out who is in charge of supervising and evaluating the output of AI.
Companies have to designate human supervisors, for example, who may check AI systems' decisions and act with corrections as needed. Without such supervision, it becomes challenging to correct mistakes or injustices brought about by AI-driven choices. Implementing a review process whereby AI recommendations are seen as ideas rather than definitive judgments can help to create responsibility frameworks by allowing human intervention room.
Platforms like StudyPro offer tools and services ranging from writing aids to more sophisticated AI-driven systems, therefore helping with tasks ranging from ethical and responsible integration of AI into decision-making processes:
Ethical AI Rules
Current ethical artificial intelligence models, notably the General Data Protection Regulation (GDPR) in Europe, provide direction on responsible AI implementation. For instance, GDPR guarantees that humans have a role in AI-driven results by including clauses allowing people to challenge choices taken by algorithms. Comparably, AI Ethics Guidelines developed by companies such as the European Commission stress the need of openness, responsibility, and justice in AI systems.
Businesses can include these ethical principles into their AI development by giving openness top priority—that is, ensuring that AI choices make sense and are understandable. This includes creating "explainable AI" models whereby users or affected people may easily understand the rationale behind an artificial intelligence's decision. Regular audits will also help companies to see whether artificial intelligence systems follow ethical guidelines, therefore guaranteeing ongoing monitoring of justice and responsibility.
Organizations can minimize the risks connected with high-stakes judgments and better negotiate the complexity of responsibility in artificial intelligence systems by combining ethical AI principles with transparent responsibility structures.
Being open to AI
Artificial intelligence needs to be open and honest about how it makes decisions so that people can trust it and hold it accountable. When AI systems are used to make important decisions in fields like banking, healthcare, or law enforcement, it's important for customers and other stakeholders to understand how those decisions are made. When there isn't enough openness, it's hard to find any mistakes, biases, or immoral behavior that might be in the system.
One of the main things that makes it hard for AI knowledge to be shared openly is the predominance of "black box" models. One example is deep learning neural networks, which are advanced machine learning systems that don't always make their decisions clear. With black box models, it can be hard to tell if the system is fair or biased because not even the engineers may be able to explain how the AI came to a certain conclusion.
Artificial intelligence that makes sense
It is the goal of the growing area of explainable AI (XAI) to make AI systems easier to understand and more open. XAI algorithms, on the other hand, are meant to give people clear reasons for the choices they make, so they can see the steps that were taken to reach a certain result. Especially in fields like health and criminal justice where the results of AI have real-world effects, this is very important.
Different groups that use and invest in XAI can get a lot of benefits.
This guide is for customers because it explains how the AI system comes up with its suggestions or judgments. This is necessary to build trust, especially when it comes to people's health, income, or freedoms.
When it comes to companies, XAI gives AI systems a way to be checked and questioned about the decisions they make when needed. Businesses can make sure that AI follows moral rules and doesn't make choices that could be dangerous by using explainable models.
Because of worries about fairness and bias, there is a large industry-wide effort to make AI systems that are easier to understand. Organizations may find research and tools like those on StudyPro platforms useful as they build and test their AI systems.
How Open-Source AI Models Are Used
When open-source artificial intelligence models are used, openness is greatly increased. When the code and methods that run AI systems are made public, anyone can look into them and figure out how they work. This includes developers, academics, and even regular people. Open-source models encourage people from different areas and subjects to work together. This lets them find possible problems, make the model work better, and make sure AI acts in an ethical way.
There are a number of benefits to using open-source AI models, such as:
- Transparency: Open-source models let you look at the whole code, which makes sure that the system doesn't have any hidden or dishonest biases.
- Cooperation: Open-source projects can benefit from comments and feedback from the community. This can help make AI systems that are more moral and better.
- Innovation: By building on existing tools that allow open access to AI models, developers can come up with new ideas while also making sure that the original models are used properly.
There are some problems with open-source AI, though. One of the main worries is safety. Since the code is available to everyone, bad people could use the models' flaws to do harm. Also, to make sure that open-source AI models are safe and moral, it might be necessary to give them more resources for ongoing upkeep and control.
Even though there are some problems, open-source AI is a strong way to encourage responsibility and openness in the creation and use of AI systems. This leads to more moral innovation in many areas.
Resolving Ethical Issues with Legislation
Governments and regulatory authorities have realized they must build frameworks addressing the ethical issues related to artificial intelligence as systems of influence and capacity keep growing. Rules are increasingly crucial for guaranteeing that artificial intelligence systems run fairly, honestly, and openly in many different sectors. Clear regulatory control helps to reduce the possibility of prejudice, injustice, and AI technology abuse, therefore possibly damaging persons and society.
Synopsis of Present and Future AI Ethics Regulations
Under construction right now, the European Union's AI Act is among the most important legislative initiatives aiming at addressing artificial intelligence ethics. The AI Act aims to create a thorough framework to control AI application in all EU industries. It seeks to classify artificial intelligence systems according to risk profiles, which range from low to high risk, and implement suitable limitations and criteria depending on these classifications. High-risk artificial intelligence systems—those applied in sectors like law enforcement or healthcare—will be subject to strict guidelines on openness, accuracy, and responsibility.
Although GDPR mostly addresses data protection, it has clauses pertaining to artificial intelligence, including the "right to explanation", wherein people may challenge conclusions rendered by AI systems. This feature of GDPR guarantees that persons affected by automated judgments have the chance to know how those decisions were taken, therefore fostering openness and justice.
Other areas, such the United States, where talks of drafting an AI Bill of Rights to direct the moral use of artificial intelligence technology have been under consideration, are seeing similar legislative initiatives. These rules seek to safeguard customers and guarantee responsible application of AI technology.
How Policies Might Guarantee Transparency, Fairness, and Responsability in AI Systems?
Laws such as the GDPR and the AI Act serve to define a set of guidelines that all artificial intelligence companies and developers have to go by. These rules can:
- Ensure Fairness: By setting standards for the types of data that AI systems use and ensuring that they are free from bias, regulations can help prevent discriminatory outcomes in AI decisions.
- Promote Accountability: Regulations can require organizations to implement clear processes for monitoring and auditing AI systems. This ensures that if something goes wrong, there are mechanisms in place to assign responsibility and correct issues.
- Foster Transparency: Mandating the use of "explainable AI" models, as well as requiring transparency in AI decision-making processes, allows users and stakeholders to understand how AI systems function and make decisions.
The Part Governments and Companies Play in Creating Ethical AI Standards
Developing, implementing, and updating laws to match the changing character of artificial intelligence technology depends critically on governments. They cannot, however, operate alone. Organizations that build and implement artificial intelligence systems must work with academic institutions, government agencies, and civil society to establish ethical AI standards that accurately represent issues and difficulties from the actual world.
Many companies are already acting pro-actively to fit new ethical standards and legal rules. Businesses are creating artificial intelligence ethics committees and internal systems to supervise the proper application of AI technology. Moreover, by interacting with government projects such as the AI Act, companies may guarantee that their AI systems comply and assist in the shaping of rules in a way that strikes ethical responsibility against innovation.
Handling ethical issues in artificial intelligence depends much on rules. They offer a structure to guarantee that artificial intelligence systems are created and applied in ways that give justice, responsibility, and openness top priority, therefore promoting a more moral and fair future for artificial intelligence.
Final Thought
Though it offers great ethical questions, artificial intelligence has the power to transform sectors, enhance quality of life, and inspire invention. Important problems such bias in artificial intelligence decision-making, lack of openness in complicated models, and the difficulties of assigning responsibility when things go wrong highlight the need of confronting artificial intelligence ethically directly. These difficulties need careful answers to guarantee that artificial intelligence systems run ethically and equitably.
Overcoming these obstacles calls for a cooperative strategy. Ethical AI design must be given top priority by developers; regulators must build thorough frameworks such as the European Union's AI Act; and society at large must remain involved in making sure that AI technologies represent shared values of justice, openness, and responsibility. Every group has a part to contribute to create artificial intelligence systems that minimize prejudice and damage and thus benefit everyone.
We must act today while artificial intelligence shapes the future. Businesses, governments, and groups of people have to cooperate to create strong ethical guidelines and behaviors. This will help to build confidence in artificial intelligence technology and open the path for a society led by fair, open, and responsible AI. Now is the moment to give ethics a priority in AI development; before these systems become so ingrained in daily life to alter.
Share this post
Leave a comment
All comments are moderated. Spammy and bot submitted comments are deleted. Please submit the comments that are helpful to others, and we'll approve your comments. A comment that includes outbound link will only be approved if the content is relevant to the topic, and has some value to our readers.
Comments (0)
No comment