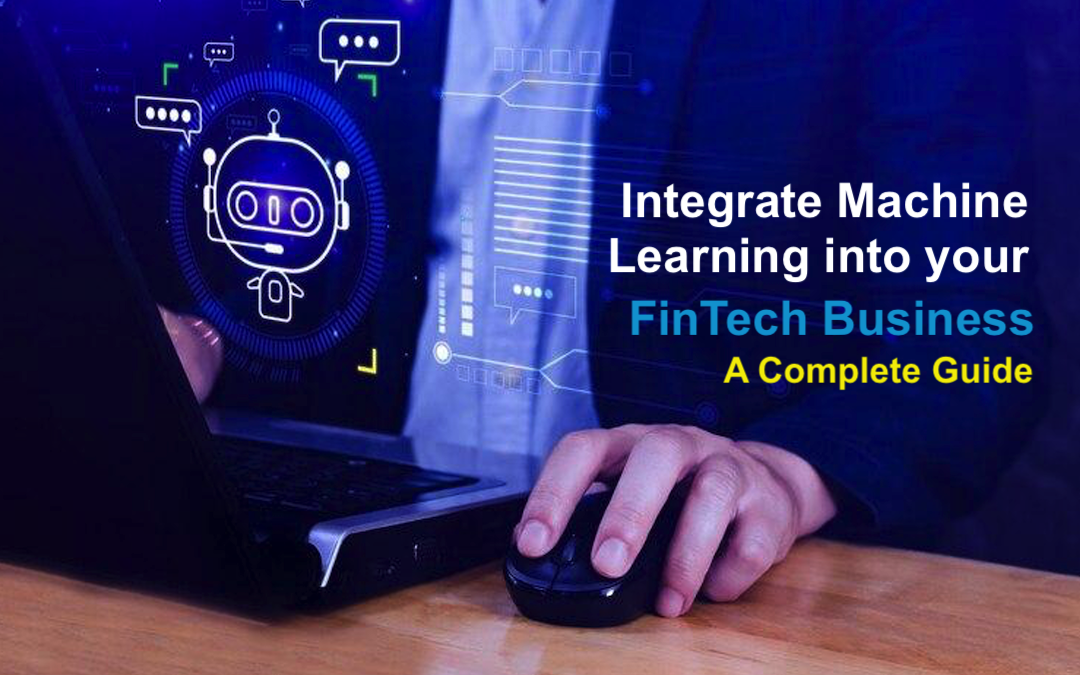
New AI and Machine Learning technologies are shaking up traditional FinTech and presenting businesses in this industry with new obstacles and possibilities. Business owners are interested in how they can use machine learning in finance to benefit their company and if investing in Machine Learning can provide a positive return on investment.
According to McKinsey's findings, approximately 55% of companies globally have implemented AI and Machine Learning in at least one aspect of their daily activities. It is expected that an increasing number of companies will prioritize innovation and incorporate machine learning into their operations in the coming years.
When examining present market data, one can observe a rising need for AI application development and machine learning services within the financial industry. Data is presented here.
- AI is utilized by 85% of financial institutions for their services.
- 52% of individuals have created financial products and services powered by artificial intelligence.
- AI in the finance sector is forecasted to achieve a value of $26.67 billion by 2025.
- AI in the FinTech sector is expected to grow to $14.41 Billion by 2024 with a compound annual growth rate of 27.1%.
How Does Machine Learning in FinTech Work?
Machine Learning is a branch of Artificial Intelligence that allows computers to learn from data, recognize patterns, and make decisions with little human involvement, enhancing their precision gradually.
Machine learning, a component of the popular tech field, has already brought about significant changes in almost every sector. This is the FinTech interpretation:
Machine Learning in FinTech focuses on improving customer service and operational procedures in FinTech and financial companies.
In the financial services sector, machine learning is commonly utilized for automating tasks, improving decision-making, and delivering customized services to customers.
Applications of Machine Learning in FinTech Solutions
Now, let's explore the technology-based applications of ML in FinTech that can help improve your business. This will demonstrate the general range of solutions to develop and implement in your day-to-day operations.
1. Forecasting Lead Scores
It is a technique to assess the probability of potential customers converting by analyzing their actions and past data. This allows for focused marketing campaigns, directing resources toward leads with the most potential.
Models that use predictive analytics to evaluate customer interactions, engagement patterns, and conversion histories to rank leads for prioritization.
2. Automated Risk Management
It includes using machine learning algorithms for real-time risk assessment, identification, and mitigation. This involves examining market trends, customer actions, and transaction information to anticipate possible risks.
Machine learning models are taught on specific data points to identify patterns that result in the appearance of risk.
3. Detection and Prevention of Fraudulent Activities
Utilizing machine learning algorithms to examine transaction data and detect irregularities that could signal potential fraud, leading to swift precautionary measures.
Detection algorithms and predictive models are used to analyze transactions to identify abnormalities and possible fraudulent activities.
4. Processing of Insurance Claims
It involves automating insurance claims processing, including data entry, document verification, and initial property assessments, to enhance efficiency and accuracy in procedures.
Document analysis using Natural Language Processing (NLP), visual damage assessments through Computer vision, and initial claim assessments using predictive models.
5. Management of Data and Processing of Documents
Automating the process of extracting, organizing, and analyzing data from extensive document collections reduces manual work and improves data accuracy.
NLP is used to extract and understand documents, while machine learning models are used to classify and analyze data.
Benefits of Using Machine Learning in FinTech
The benefits of using generative AI in FinTech, along with the corresponding AI solutions, offer value-adding advantages.
1. Reduction in expenses
ML-based process automation solutions in the FinTech industry minimize manual labor requirements to some degree. This leads to considerable cost reductions and the streamlined management system needed for the company.
The average percentage of all operation costs in FinTech attributed to staff costs is 27.8%. Just think about the potential cost savings that can be achieved through optimizations in expenses.
2. Complete adherence to regulations
ML aids FinTech software development firms in meeting data security standards such as HIPAA and GDPR by using automated tools for compliance monitoring and reporting. Earning customer loyalty and trust can prevent non-compliance penalties of up to 4% of annual revenue.
3. Enhanced Security
Artificial intelligence-powered systems for detecting fraud, anomalies, and monitoring transactions can pinpoint questionable behaviors. This results in safeguarding FinTech companies and their customers against possible dangers.
4. Better customer experience
Chatbots and personalized financial advice systems allow for round-the-clock customer service and customized product options. This leads to a better and more enjoyable user experience, effectively fulfilling their needs.
5. Strategic decision making
Forecasting analysis and analyzing large amounts of data offer information on market patterns, consumer actions, and possible monetary hazards. This results in more intelligent, data-informed strategic planning.
Challenges Faced While Implementing Machine Learning in FinTech
To fully appreciate both the advantages and disadvantages of using ML in FinTech, it is essential to grasp the typical obstacles encountered in ML endeavors. Below are a few examples.
Data accessibility and Quality Issues
Machine learning technology needs abundant, top-notch, varied, and inclusive data to learn well and produce precise predictions. In FinTech, it can be difficult to acquire this information because of discrepancies, errors, and missing pieces in financial data sources.
Expensive costs for implementation
Creating and implementing ML solutions can be expensive, requiring investment in data acquisition, model building, computing resources, and talent recruitment. Furthermore, the continuous upkeep and revisions of the model also add to the expenses.
Concerns regarding ethics and bias
Machine learning algorithms have the potential to unknowingly acquire and sustain biases from the data they are trained on, resulting in unjust or discriminatory results. This is especially troublesome in FinTech apps like credit scoring, as biased algorithms may lead to unjust denial of services for specific groups.
Conclusion
Currently, machine learning is popular in the banking and finance sector. With the majority of companies incorporating smart technology into their operations and many businesses opting for comprehensive custom machine learning solutions, it can be confidently stated that projects based on this technology show great potential as investments.
Related Posts
Share this post
Leave a comment
All comments are moderated. Spammy and bot submitted comments are deleted. Please submit the comments that are helpful to others, and we'll approve your comments. A comment that includes outbound link will only be approved if the content is relevant to the topic, and has some value to our readers.
Comments (0)
No comment