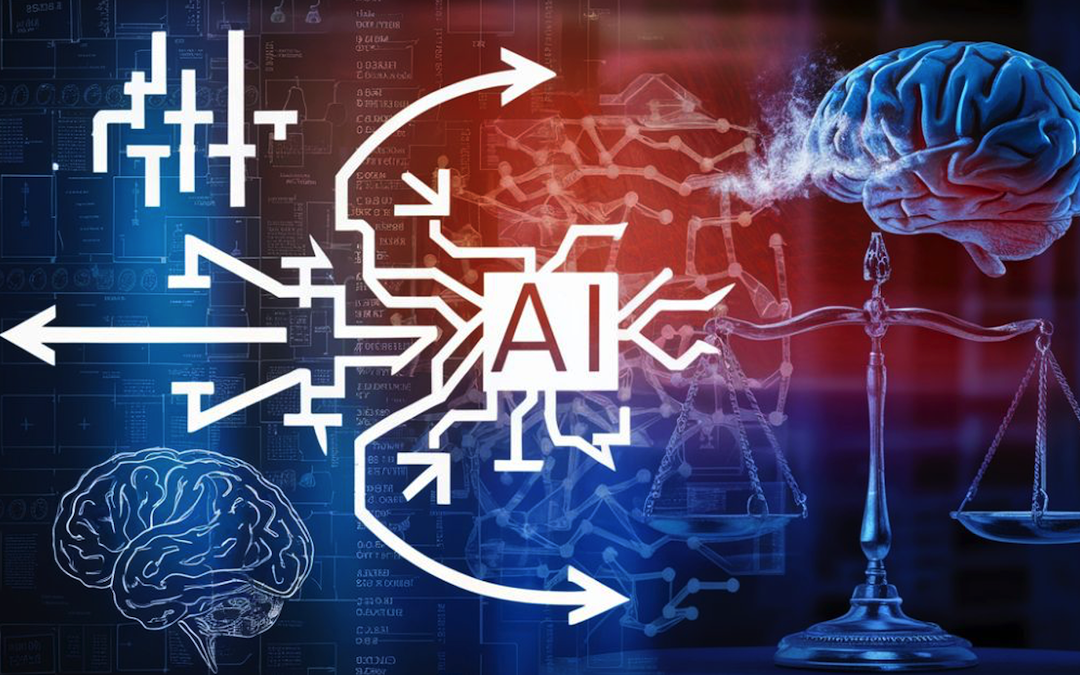
AI bias refers to situations in which artificial intelligence applications use inaccurate information or math that is biased or unjustly.
When artificial intelligence systems fail to take into account flawed data or algorithms in selecting choices that are unfair or discriminatory it is referred to as AI Bias in data-driven decisions. Unequal treatment or perpetuating discrimination are only a few among many ethical dilemmas raised by this type of bias. To create equitable and just AI systems for all persons, this issue must be addressed by understanding and tackling AI Bias.
The root of AI bias is in data-driven decision-making where AI systems make biased choices either due to a lack of fairness in their data or algorithms. When AI shows bias, it becomes a matter of ethics since there might be cases of favoritism and inequality in offering chances.
Understanding AI Bias
AI Bias occurs when AI systems produce discriminatory outputs due to skewed data or faulty algorithms. This can result in inequality against specific groups. A case in point, a tool for hiring using AI algorithms may favor a particular gender. It remains essential to appreciate AI Bias for the sake of equity maintenance.
Therefore, diversity and representation of the data that the AI trains on should be ensured. Designing fair algorithms will help decrease biases in them while ensuring validation throughout by regularly checking for such distortions.
AI bias concerns regarding ethics are critical. Partial AI might cause skewed opportunities. Thus, we talk about fairness and justice here. Organizations require an understanding of how their constructs operate. It is also their responsibility to answer for outcomes caused by bias. Having policies and principles on ethics can prevent others from being harmed. For trust in these technologies to be gained, they need to do this.
Sources of AI Bias
- Historical Bias in Data: Past data may reflect societal prejudices, influencing AI outcomes.
- Lack of Diverse Data: Bad artificial intelligence decisions are caused by limited or biased data.
- Algorithm Design Choices: Developers' biases can unintentionally shape AI behavior.
- Bias in Data Processing: Errors during data cleaning and preparation can introduce bias.
- Feedback Loops: AI systems are made better and continuous existing biases with their biased outcomes.
- Incomplete Data: AI decisions can be biased as a result of the absence or incorrect data.
- Cultural Bias: AI trained on data from one culture may not perform fairly across diverse groups.
Impact of AI Bias
Impact of AI Bias | Ethical Considerations |
---|---|
Discrimination | Unfair treatment of certain groups, leading to inequality |
Loss of Trust | Biased outcomes are responsible for why AI has so many doubters. |
Reduced Fairness | Compromised fairness in decisions affecting individuals' lives |
Inequitable Opportunities | Disparity in the allotment of resources and opportunities. |
Legal Ramifications | Potential violations of laws and regulations |
Social Harm | Promoting stereotypes and social rifts |
Accountability Issues | Difficulty in holding AI developers and users responsible |
Identifying AI Bias
One of the things about identifying artificial intelligence bias is to recognize circumstances in which erroneous data or prejudiced algorithms lead to unjust outcomes. Bias in AI technologies can cause continuing discrimination and inflict harm on individuals or groups, hence the need for moral considerations.
Determining if there exists a bias requires examining the processes used in making decisions by these systems and looking at their output keenly. Statistical analysis methods and bias auditing tools are some of the ways that can help to uncover concealed biases.
Artificial intelligence is biased according to ethics because identification should be transparent and responsible. Disclosures of possible biases by participants during training will be important. Unbiasedness can be maintained through continual auditing including monitoring so long as bias is controlled over time. Before spreading, there should be detection and eradication of prejudice among organizations who wish to comply with ethics while using technological advancements based upon trust building among them and AI developers.
Mitigating AI Bias
To reduce unfair outcomes from AI systems, measures targeting a reduction in AI bias are needed. The results of an unfair AI system can be prejudiced and inequities hence there is a great necessity for thinking about ethics when we speak in regards to biased artificial intelligence. One among them is having different types of content that approximate each other while training personal AI models.
Developers must create algorithms that focus on equity and transparency. Consistent appraisals will aid in detecting and correcting prejudices before making decisions by them. To realize this goal, ethical guidelines and regulations should be implemented to ensure accountability as well as promote unbiased AI development.
Analyze and address ethical issues in devising robust mitigation strategies that are fair, transparent, and trustworthy to all concerned parties, including the users and stakeholders.
Conclusion
There are occasions where the decisions that are driven by data lead to biased AI outputs from flawed data or non-neutral algorithms at least sometimes. Biased AI is an ethical concern since it can lead to further inequality and erode trust in technology. Pre-emptive solutions such as various data sources, algorithmic openness, and constant audits need to be laid down against AI bias.
To create fair and just AI systems that comply with ethical standards it is important to grasp and address AI Bias first. Prioritizing ethics while enforcing strict oversight allows us to leverage AI’s benefits without significantly reducing or eliminating the harmful consequences it may have on society. To ensure fair and responsible application of technology in decision-making processes, ethical issues should prevail over AI development stages.
Comments (0)
No comment