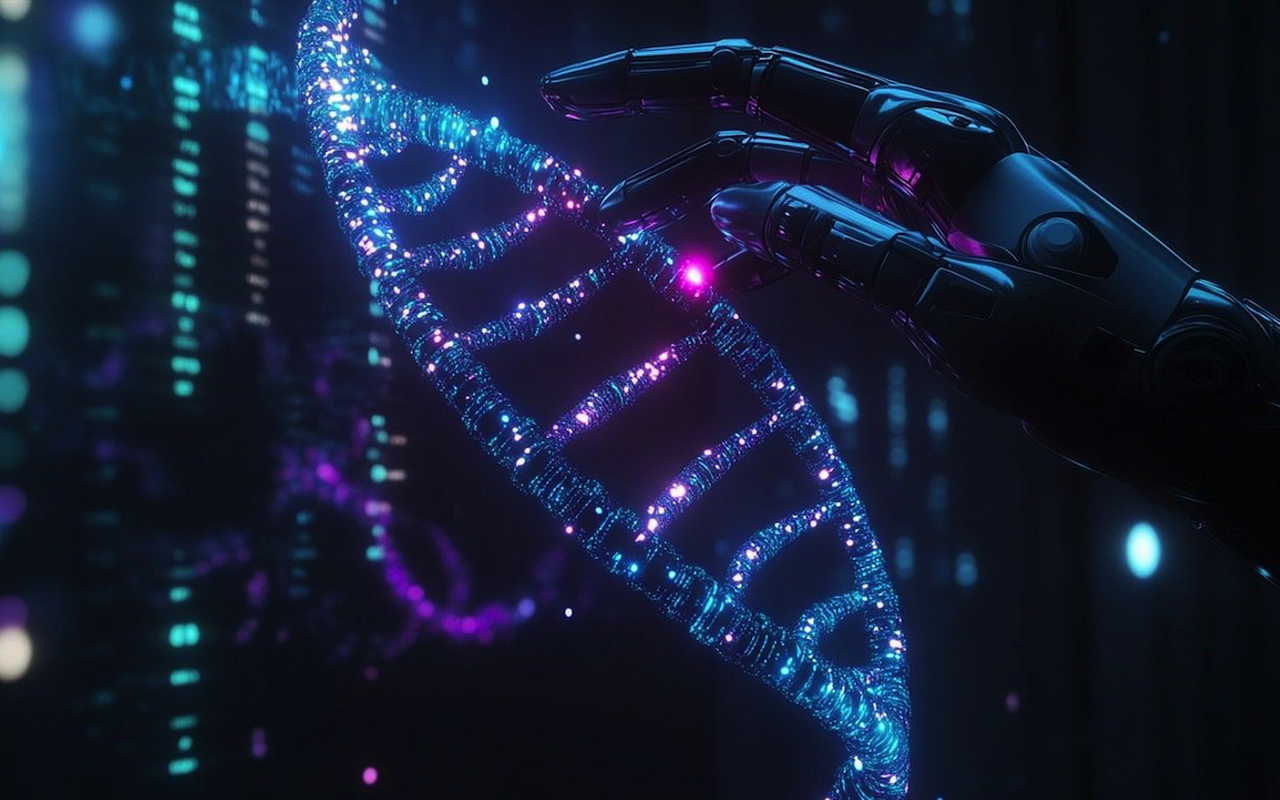
For AI models to perform effectively, they require well-labeled, high-quality data, which is where data annotation services come in, to ready datasets for machine learning. This is important for image recognition, NLP, and autonomous systems. However, the price can differ due to factors such as complexity, total amount, and workers employed.
Understanding these budget drivers helps businesses budget effectively. This way, they can choose the right data labeling service without overspending. This article explains important pricing factors. It also shares strategies to cut costs while keeping accuracy.
Core Factors That Impact Data Annotation Costs
The pricing of data annotation services shifts. It depends on data type, project size, and quality of annotation. Here’s what influences pricing the most.
Type and Complexity of Data
Different data formats require different levels of effort:
- Text annotation (e.g., sentiment analysis) is simpler and costs less.
- Image and video labeling (e.g., object detection, segmentation) takes more time and expertise, making it more expensive.
- Audio annotation, like speech-to-text, is more expensive. This is because it requires manual transcription and identifying speakers.
AI data labeling for facial recognition is more expensive than basic object detection.
Volume and Scalability
Larger projects often get lower per-unit rates. However, they need:
- Efficient tools to speed up annotation.
- Trained annotators to maintain quality.
- Scalable systems to handle high data loads.
Long-term contracts can reduce spending while ensuring consistent results.
Annotation Detail and Quality
The more detailed the annotation, the higher expenses. Key factors include:
- Annotation depth: Basic tags cost less than detailed segmentations.
- Quality control: Multistep validation and expert reviews increase spending.
- Revisions: Corrections and rework add to the budget.
Human vs. Automated Annotation
Automation can lower costs, but it’s not always accurate.
- Rule-based automation works for simple tasks but struggles with complex data.
- AI-assisted annotation is faster, but often needs human review.
- Manual annotation is the most precise but also the most expensive.
Choosing between human and automated data labeling tools depends on budget, accuracy needs, and project size.
Workforce and Geographic Pricing Variations
Labor costs vary depending on who does the annotation and where they’re located. Choosing in-house teams or outsourcing matters. Picking a provider in the right area can also affect the budget.
In-House vs. Outsourced Teams
Building an in-house team gives full control over quality but comes with higher costs:
- Hiring and training: Recruiting skilled annotators takes time and resources.
- Infrastructure: Companies need data labeling tools, storage, and security measures.
- Scaling challenges: Expanding the team for large projects adds expenses.
Cost-Efficient Benefits of Outsourcing
Outsourcing to a data labeling service is often more cost-effective:
- Lower labor costs: No need for in-house salaries and benefits.
- Faster turnaround: Dedicated teams work around the clock.
- Access to expertise: Providers specialize in different annotation types.
Companies handling long-term or highly sensitive projects may prefer in-house teams, while outsourcing works well for scalable, budget-friendly solutions.
Geographic Cost Disparities
Annotation costs vary by region due to differences in labor rates and expertise:
Region | Average Cost per Hour ($USD) | Common Use Cases |
---|---|---|
North America | 20 – 50 | High-quality, sensitive data |
Western Europe | 15 – 40 | Specialized annotation, GDPR-compliant projects |
Eastern Europe | 8 – 20 | Cost-effective, skilled annotators |
South Asia | 5 – 15 | Large-scale, budget-friendly annotation |
Southeast Asia | 4 – 12 | Affordable, scalable workforce |
While lower-cost regions offer savings, quality can vary. Businesses should evaluate data collection services by checking their accuracy rates, past projects, and adherence to security standards.
Tools, Infrastructure, and Security Considerations
The right tools and security measures can significantly impact the cost of data annotation services. Free or low-cost solutions may save money upfront, but they can slow down projects or compromise quality. Security and compliance requirements can also add expenses.
Impact of Annotation Tools and Platforms
Data labeling tools range from free open-source software to enterprise-grade platforms. The choice affects both budget and efficiency:
- Free tools (e.g., CVAT, LabelImg) work for small projects but may lack automation features.
- Commercial platforms like Labelbox and Scale AI provide AI-assisted annotation and quality control. However, they charge licensing fees.
- Custom-built solutions provide tailored workflows but require development costs.
For high-volume AI data labeling, using advanced tools can help speed up work. They also boost accuracy, which cuts down on expensive revisions.
Data Security and Compliance Costs
Costs rise due to security and compliance needs, especially in fields such as healthcare and finance. Key factors include:
- Data encryption: Protecting sensitive information increases processing costs.
- Access control: Secure workflows require authentication and role-based permissions.
- Regulatory compliance: GDPR, HIPAA, and other regulations add legal and operational costs.
Using a data labeling service with strict security protocols protects your data, but it might raise expenses.
Pricing Models and Cost Estimation
Different pricing structures affect how businesses pay for data annotation services. Choosing the right model can help control costs while ensuring quality.
Common Pricing Structures
Annotation providers use different pricing models based on project needs:
- Per-hour: Best for complex tasks where time varies. Rates depend on workforce location and expertise.
- Per-label: Suitable for large datasets with simple annotations. Costs vary based on annotation type.
- Per-dataset: Fixed pricing for full datasets, ideal for projects with clear scope.
- Project-based: Custom pricing for big, high-precision projects. This includes quality control and several review cycles.
Businesses should compare models to see which aligns with their data volume, accuracy needs, and budget.
Hidden Costs to Consider
Beyond the base price, some costs can add up:
- Quality control: Additional review layers increase expenses.
- Revisions: Errors or inconsistencies may require rework.
- Training annotators: Specialized tasks may need onboarding time.
Planning for these factors helps avoid unexpected expenses and keeps data labeling projects on track.
How to Optimize Data Annotation Costs Without Compromising Quality
Reducing costs doesn’t mean sacrificing quality. Good planning and strong partnerships can make data annotation services affordable and accurate.
Choosing the Right Partner
Selecting a reliable provider ensures efficiency and minimizes hidden costs. Consider:
- Expertise: Look for experience in your industry and annotation type.
- Quality assurance: Providers with multi-step validation prevent costly rework.
- Scalability: A team that adapts to your needs avoids delays and budget overruns.
A trusted data labeling partner can offer cost savings over the long haul by reducing errors and refining operations.
Balancing Budget and Quality
Cutting costs the right way prevents issues down the line. Strategies include:
- Using AI-assisted tools: Automation speeds up simple tasks, while humans handle complex ones.
- Preprocessing data: Cleaning and structuring data before annotation reduces workload.
- Batch processing: Testing a small sample first prevents large-scale errors.
Businesses that plan ahead and use data labeling tools well can save money and keep accuracy.
Conclusion
The cost of data annotation services varies. It depends on factors like data complexity, project size, workforce choice, and security needs. Choosing the right pricing model and partner can help balance cost and quality.
Businesses can cut costs by using data labeling tools, improving workflows, and considering outsourcing. They can do this while still keeping accuracy intact. Careful planning ensures efficient, scalable annotation that supports AI development without unnecessary costs.
Share this post
Leave a comment
All comments are moderated. Spammy and bot submitted comments are deleted. Please submit the comments that are helpful to others, and we'll approve your comments. A comment that includes outbound link will only be approved if the content is relevant to the topic, and has some value to our readers.
Comments (0)
No comment